Last post, we looked at the intuition and the formulation of Level Set Method. It’s useful to create a physical simulation like front propagation, e.g. wave simulation, wildfire simulation, or gas simulation. In this post, we are going to see into Level Set Method application in Computer Vision, to solve image segmentation problem.
Image Segmentation with Level Set Method
Recall that the Level Set PDE that we have derived in the last post is as follows:
Here,
As we want to segment an image, then we need to derive
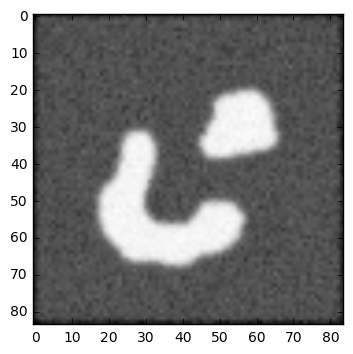
As
One way to do it is obviously derive our
So, we could implement it with the code below:
import numpy as npimport scipy.ndimageimport scipy.signalimport matplotlib.pyplot as pltfrom skimage import color, io
def grad(x): return np.array(np.gradient(x))
def norm(x, axis=0): return np.sqrt(np.sum(np.square(x), axis=axis))
def stopping_fun(x): return 1. / (1. + norm(grad(x))**2)
img = io.imread('twoObj.bmp')img = color.rgb2gray(img)img = img - np.mean(img)
# Smooth the image to reduce noise and separation between noise and edge becomes clearimg_smooth = scipy.ndimage.filters.gaussian_filter(img, sigma)
F = stopping_fun(img_smooth)
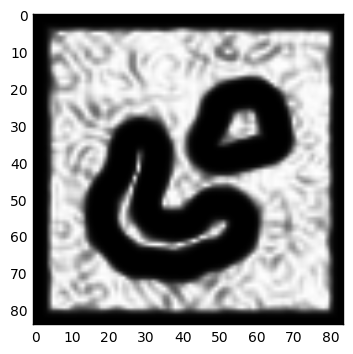
So that’s it, we have our
def default_phi(x): # Initialize surface phi at the border (5px from the border) of the image # i.e. 1 outside the curve, and -1 inside the curve phi = np.ones(x.shape[:2]) phi[5:-5, 5:-5] = -1. return phi
dt = 1.
for i in range(n_iter): dphi = grad(phi) dphi_norm = norm(dphi) dphi_t = F * dphi_norm phi = phi + dt * dphi_t
And here’s the segmentation result after several iteration:
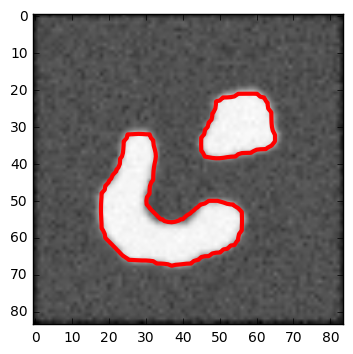
This is the naive method for Level Set image segmentation. We could do better by using more complicated formulation for
Geodesic Active Contour
In Geodesic Active Contour (GAC) formulation of Level Set Method, we define the force as:
The first term is the smoothing term, it moves the curve into the direction of its curvature. The second term is the balloon term, controlling the speed of the curve propagation with parameter
def curvature(f): fy, fx = grad(f) norm = np.sqrt(fx**2 + fy**2) Nx = fx / (norm + 1e-8) Ny = fy / (norm + 1e-8) return div(Nx, Ny)
def div(fx, fy): fyy, fyx = grad(fy) fxy, fxx = grad(fx) return fxx + fyy
def dot(x, y, axis=0): return np.sum(x * y, axis=axis)
v = 1.dt = 1.
g = stopping_fun(img_smooth, alpha)dg = grad(g)
for i in range(n_iter): dphi = grad(phi) dphi_norm = norm(dphi) kappa = curvature(phi)
smoothing = g * kappa * dphi_norm balloon = g * dphi_norm * v attachment = dot(dphi, dg)
dphi_t = smoothing + balloon + attachment
phi = phi + dt * dphi_t
Finally, here’s the result of using GAC formulation of Level Set Method for segmenting the same image above:
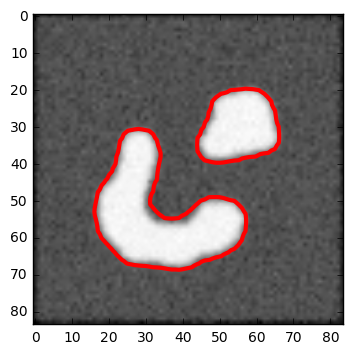
Notice that qualitatively the segmentation result is better than before, i.e. smoother and better fit.
Conclusion
In this post we looked into the application of Level Set Method on Computer Vision problem, that is image segmentation. We saw the intuition on applying it for image segmentation. We also saw the example of implementation of it. Finally we saw the more complicated formulation, i.e. GAC, for better segmentation.
References
- Richard Szeliski. 2010. Computer Vision: Algorithms and Applications (1st ed.). Springer-Verlag New York, Inc., New York, NY, USA.