Generative Adversarial Networks (GAN) is one of the most exciting generative models in recent years. The idea behind it is to learn generative distribution of data through two-player minimax game, i.e. the objective is to find the Nash Equilibrium. For more about the intuition and implementation of GAN, please see my previous post about GAN and CGAN.
Note, the TensorFlow and Pytorch code could be found here: https://github.com/wiseodd/generative-models.
One natural extension of GAN is to learn a conditional generative distribution. The conditional could be anything, e.g. class label or even another image.
However, we need to provide those conditionals manually, somewhat similar to supervised learning. InfoGAN, therefore, attempted to make this conditional learned automatically, instead of telling GAN what that is.
InfoGAN intuition
Recall, in CGAN, the generator network has an additional parameter:
In CGAN,
As
So how does InfoGAN do that? This is when information theory takes part.
In information theory, if we want to express the knowledge about something if we know something else, we could use mutual information. So, if we maximize mutual information, we could find something that could contribute to the knowledge of another something the most. In our case, we want to maximize the knowledge about our conditional variable
The InfoGAN mutual information loss is formulated as follows:
where
This mutual information term fits in the overall GAN loss as a regularization:
where
InfoGAN training
During training, we provide a prior
The training process is similar for discriminator net
- instead of
, we use discriminator as in vanilla GAN: , i.e. unconditional discriminator, - instead of feeding observed data for the
, e.g. labels, into , we sample from prior .
In addition to
InfoGAN implementation in TensorFlow
The implementation for vanilla and conditional GAN could be found here: GAN, CGAN. We will focus on the additional implementation for InfoGAN in this section.
We will implement InfoGAN for MNIST data, with
As seen in the loss function of InfoGAN, we need one additional network,
Q_W1 = tf.Variable(xavier_init([784, 128]))Q_b1 = tf.Variable(tf.zeros(shape=[128]))
Q_W2 = tf.Variable(xavier_init([128, 10]))Q_b2 = tf.Variable(tf.zeros(shape=[10]))
theta_Q = [Q_W1, Q_W2, Q_b1, Q_b2]
def Q(x): Q_h1 = tf.nn.relu(tf.matmul(x, Q_W1) + Q_b1) Q_prob = tf.nn.softmax(tf.matmul(Q_h1, Q_W2) + Q_b2) return Q_prob
that is, we model
Next, we specify our prior:
def sample_c(m): return np.random.multinomial(1, 10*[0.1], size=m)
which is a categorical distribution, with equal probability for each of the ten elements.
As training
G_sample = generator(Z, c)Q_c_given_x = Q(G_sample)
during runtime, we will populate sample_c()
.
Having all ingredients in hands, we could compute the mutual information term, which is the conditional entropy of the prior and our variational distribution, plus the entropy of our prior. Observe however, our prior is a fixed distribution, thus the entropy will be constant and can be left out.
cond_ent = tf.reduce_mean(-tf.reduce_sum(tf.log(Q_c_given_x + 1e-8) \* c, 1))Q_loss = cond_ent
Then, we optimize both
Q_solver = tf.train.AdamOptimizer().minimize(Q_loss, var_list=theta_G + theta_Q)
We initialized the training as follows:
for it in range(1000000): """ Sample X_real, z, and c from priors """ X_mb, _ = mnist.train.next_batch(mb_size) Z_noise = sample_Z(mb_size, Z_dim) c_noise = sample_c(mb_size)
""" Optimize D """ _, D_loss_curr = sess.run([D_solver, D_loss], feed_dict={X: X_mb, Z: Z_noise, c: c_noise})
""" Optimize G """ _, G_loss_curr = sess.run([G_solver, G_loss], feed_dict={Z: Z_noise, c: c_noise})
""" Optimize Q """ sess.run([Q_solver], feed_dict={Z: Z_noise, c: c_noise})
After training, we could see what property our prior c = [0, 0, 1, 0, 0, 0, 0, 0, 0, 0]
, we might get this:
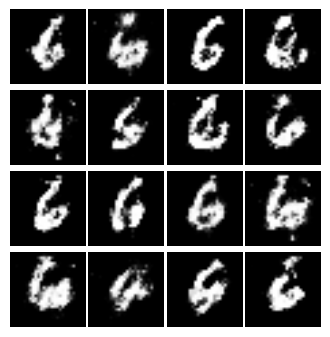
Note, naturally, there is no guarantee on the ordering of
We could try different values for
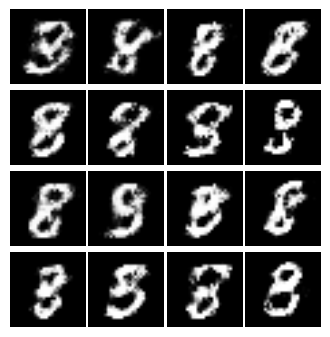
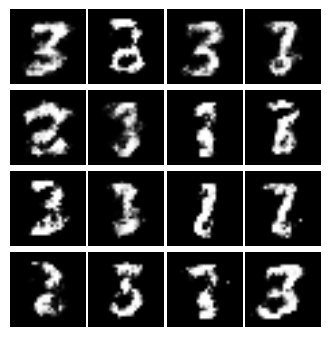
We could see that our implementation of InfoGAN could capture the conditional variable, which in this case is the labels, in unsupervised manner.
Conclusion
In this post we learned the intuition of InfoGAN: a conditional GAN trained in unsupervised manner.
We saw that InfoGAN learns to map the prior
We also implemented InfoGAN in TensorFlow, which as we saw, it is a simple modification from the original GAN and CGAN.
The full code, both TensorFlow and Pytorch implementations are available in: https://github.com/wiseodd/generative-models.
References
- Chen, Xi, et al. “Infogan: Interpretable representation learning by information maximizing generative adversarial nets.” Advances in Neural Information Processing Systems. 2016.