/ 6 min read
Implementing Dropout in Neural Net
Dropout is one of the recent advancement in Deep Learning that enables us to train deeper and deeper network. Essentially, Dropout act as a regularization, and what it does is to make the network less prone to overfitting.
As we already know, the deeper the network is, the more parameter it has. For example, VGGNet from ImageNet competition 2014, has some 148 million parameters. That’s a lot. With that many parameters, the network could easily overfit, especially with small dataset.
Enter Dropout.
In training phase, with Dropout, at each hidden layer, with probability p
, we kill the neuron. What it means by ‘kill’ is to set the neuron to 0. As neural net is a collection multiplicative operations, then those 0 neuron won’t propagate anything to the rest of the network.
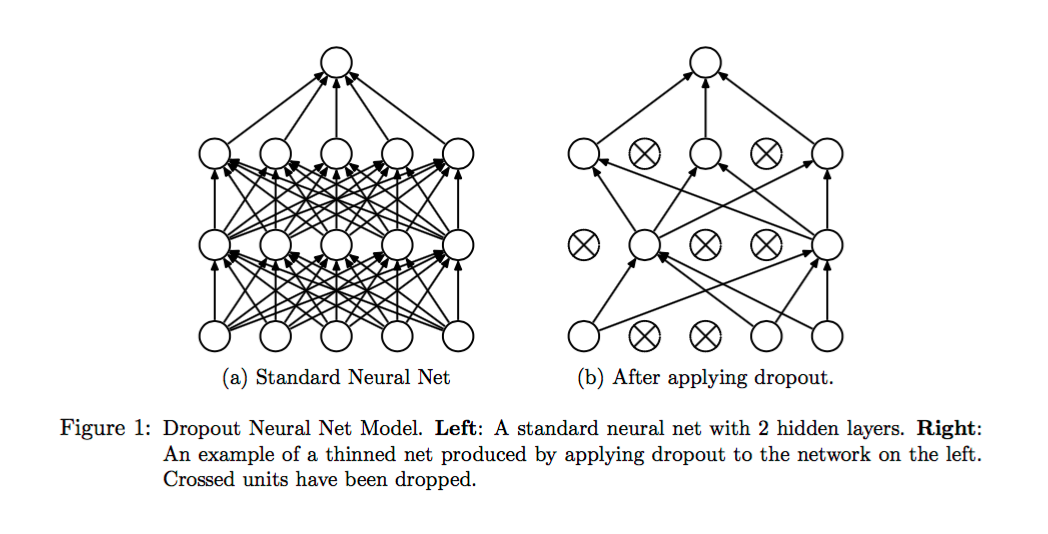
Let n
be the number of neuron in a hidden layer, then the expectation of the number of neuron to be active at each Dropout is p*n
, as we sample the neurons uniformly with probability p
. Concretely, if we have 1024 neurons in hidden layer, if we set p = 0.5
, then we can expect that only half of the neurons (512) would be active at each given time.
Because we force the network to train with only random p*n
of neurons, then intuitively, we force it to learn the data with different kind of neurons subset. The only way the network could perform the best is to adapt to that constraint, and learn the more general representation of the data.
It’s easy to remember things when the network has a lot of parameters (overfit), but it’s hard to remember things when effectively the network only has so many parameters to work with. Hence, the network must learn to generalize more to get the same performance as remembering things.
So, that’s why Dropout will increase the test time performance: it improves generalization and reduce the risk of overfitting.
Let’s see the concrete code for Dropout:
# Dropout trainingu1 = np.random.binomial(1, p, size=h1.shape)h1 *= u1
First, we sample an array of independent Bernoulli Distribution, which is just a collection of zero or one to indicate whether we kill the neuron or not. For example, the value of u1
would be np.array([1, 0, 0, 1, 1, 0, 1, 0])
. Then, if we multiply our hidden layer with this array, what we get is the originial value of the neuron if the array element is 1, and 0 if the array element is also 0.
For example, after Dropout, we need to do h2 = np.dot(h1, W2)
, which is a multiplication operation. What is zero times x? It’s zero. Then the subsequent multiplications would be also zero. That’s why those 0 neurons won’t contribute anything to the rest of the propagation.
Now, because we’re only using p*n
of the neurons, the output then has the expectation of p*x
, if x
is the expected output if we use all the neurons (without Dropout).
As we don’t use Dropout in test time, then the expected output of the layer is x
. That doesn’t match with the training phase. What we need to do is to make it matches the training phase expectation, so we scale the layer output with p
.
# Test time forward passh1 = X_train @ W1 + b1h1[h1 < 0] = 0
# Scale the hidden layer with ph1 *= p
In practice, it’s better to simplify things. It’s cumbersome to maintain codes in two places. So, we move that scaling into the Dropout training itself.
# Dropout training, notice the scaling of 1/pu1 = np.random.binomial(1, p, size=h1.shape) / ph1 *= u1
With that code, we essentially make the expectation of layer output to be x
instead of px
, because we scale it back with 1/p
. Hence in the test time, we don’t need to do anything as the expected output of the layer is the same.
Dropout backprop
During the backprop, what we need to do is just to consider the Dropout. The killed neurons don’t contribute anything to the network, so we won’t flow the gradient through them.
dh1 *= u1
For full example, please refer to: https://github.com/wiseodd/hipsternet/blob/master/hipsternet/neuralnet.py.
Test and Comparison
Test time! But first, let’s declare what kind of network we will use for testing.
def make_network(D, C, H=100): model = dict( W1=np.random.randn(D, H) / np.sqrt(D / 2.), W2=np.random.randn(H, H) / np.sqrt(H / 2.), W3=np.random.randn(H, C) / np.sqrt(H / 2.), b1=np.zeros((1, H)), b2=np.zeros((1, H)), b3=np.zeros((1, C)) )
return model
model = nn.make_network(D, C, H=256)
We’re using three layers network with 256 neurons in each hidden layer. The weights are initialized using Xavier divided by 2, as proposed by He, et al, 2015. The data used are MNIST data with 55000 training data and 10000 test data. The optimization algorithm used is RMSprop with 1000 iterations, repeated 5 times and the test accuracy is averaged.
# Without Dropoutrmsprop => mean accuracy: 0.9640, std: 0.0071
# With Dropoutrmsprop => mean accuracy: 0.9692, std: 0.0006
Looking at the result, model which use Dropout yield a better accuracy across the test set. The difference of 0.005 might be negligible, but considering we have 10000 test data, that’s quite a bit.
The standard deviation of the test tells different story though. It seems that the network that uses Dropout for training perform consistently better during test time. Compare it to the non-Dropout network, it’s an order of magnitude worse in term of consistency. We can see this when comparing the standard deviation: 0.0006 vs 0.0071.
However, when we look at the convergence of the network during training, it seems that non-Dropout network converge better and faster. Here, we could see at the loss at every 100 iterations.
# Without DropoutIter-100 loss: 0.7141623845363005Iter-200 loss: 0.5242217596766273Iter-300 loss: 0.37112553379849605Iter-400 loss: 0.38909851968506987Iter-500 loss: 0.25597567938296006Iter-600 loss: 0.30120887447912315Iter-700 loss: 0.24511170871806906Iter-800 loss: 0.23164132479234184Iter-900 loss: 0.18410249409092522Iter-1000 loss: 0.21936521877677104
# With DropoutIter-100 loss: 0.8993988029028332Iter-200 loss: 0.761899148472519Iter-300 loss: 0.6472785867227253Iter-400 loss: 0.4277826704557144Iter-500 loss: 0.48772494633262575Iter-600 loss: 0.35737694600178316Iter-700 loss: 0.3650990861796465Iter-800 loss: 0.30701377662168766Iter-900 loss: 0.2754936912501326Iter-1000 loss: 0.3182353552441539
This indicates that network without Dropout performs better at the training phase while Dropout network perform worse. The table is turned at the test time, Dropout network is not just perform better, but consistenly better. One could interpret this as the sign of overfitting. So, really, we could see that Dropout regularize our network and make it more robust to overfitting.
Conclusion
We look at one of the driving force of the recent advancement of Deep Learning: Dropout. It’s a relatively new technique but already made a very big impact in the field. Dropout act as regularizer by stochastically kill neurons in hidden layers. This in turn force the network to generalize more.
We also implement Dropout in our model. Implementing Dropout in our neural net model is just a matter of several lines of code. We found that it’s a very simple method to implement.
We then compare the Dropout network with non Dropout network. The result is nice: Dropout network performs consistenly better in test time compared to the non Dropout Network.
To see more about, check my full example in my Github page: https://github.com/wiseodd/hipsternet.