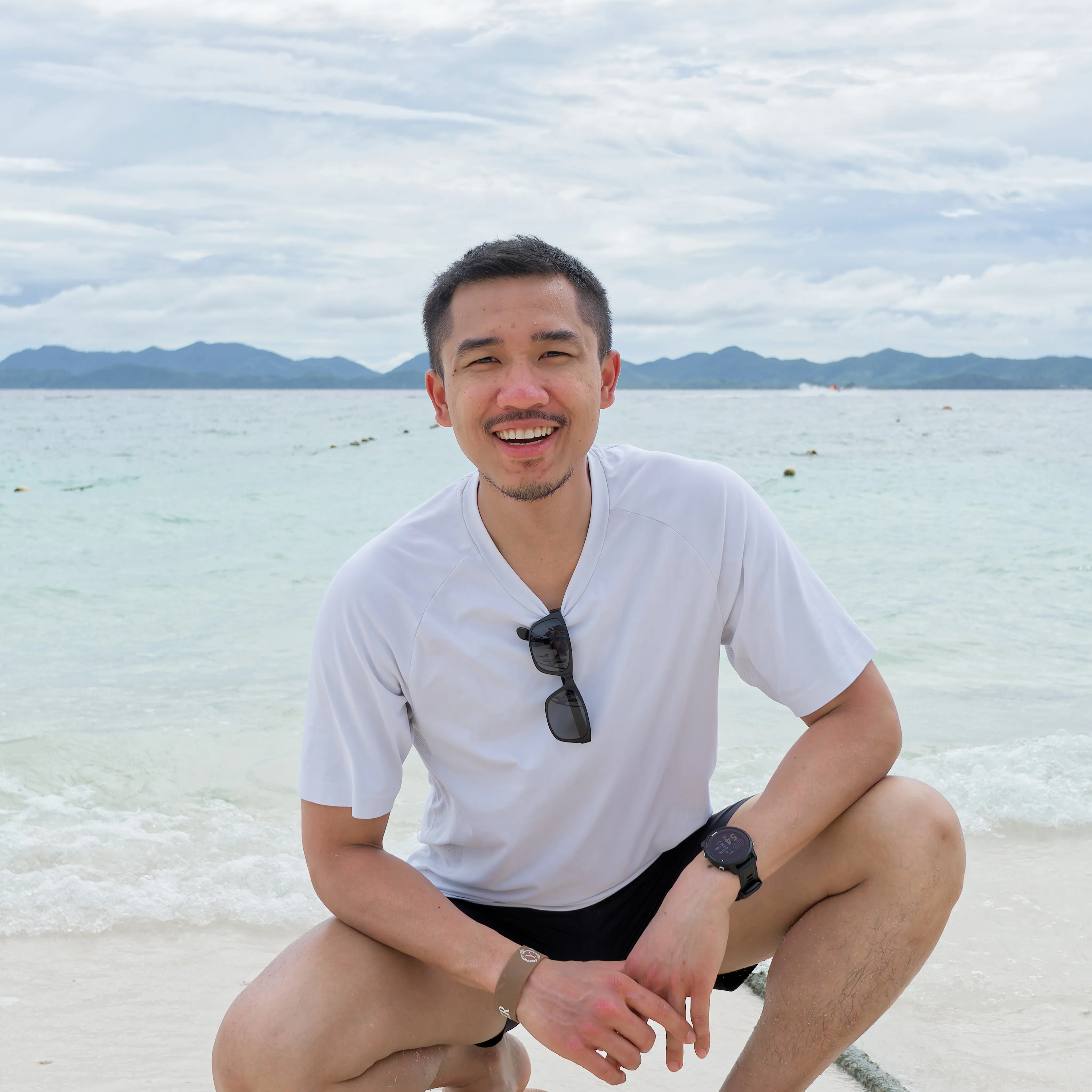
Agustinus Kristiadi
About
Agustinus Kristiadi is a postdoctoral fellow at the Vector Institute in Toronto, and previously obtained his PhD from the University of Tuebingen in Germany. His research interests are in probabilistic methods for large-scale neural networks, decision-making under uncertainty, and their applications in broader scientific fields such as chemistry. His work has been recognized in the form of best PhD thesis award and multiple spotlight papers from flagship machine learning conferences. His contributions to the scientific society include mentoring underrepresented students and actively contributing to the open-source community.
Interests
News
- I will be in Singapore between 28 and 30 April, inclusive. Feel free to reach out! I am also organizing the AABI 2025 symposium on the 29th.
- I gave a talk at UBC's first-year seminar in science (SCIE 113). I talked about the high-level intuition of probabilistic decision-making algorithms and their impacts in making the world a better place.
- Hello, world! I'm starting this news section!